AUTOMOTIVE DIVISION
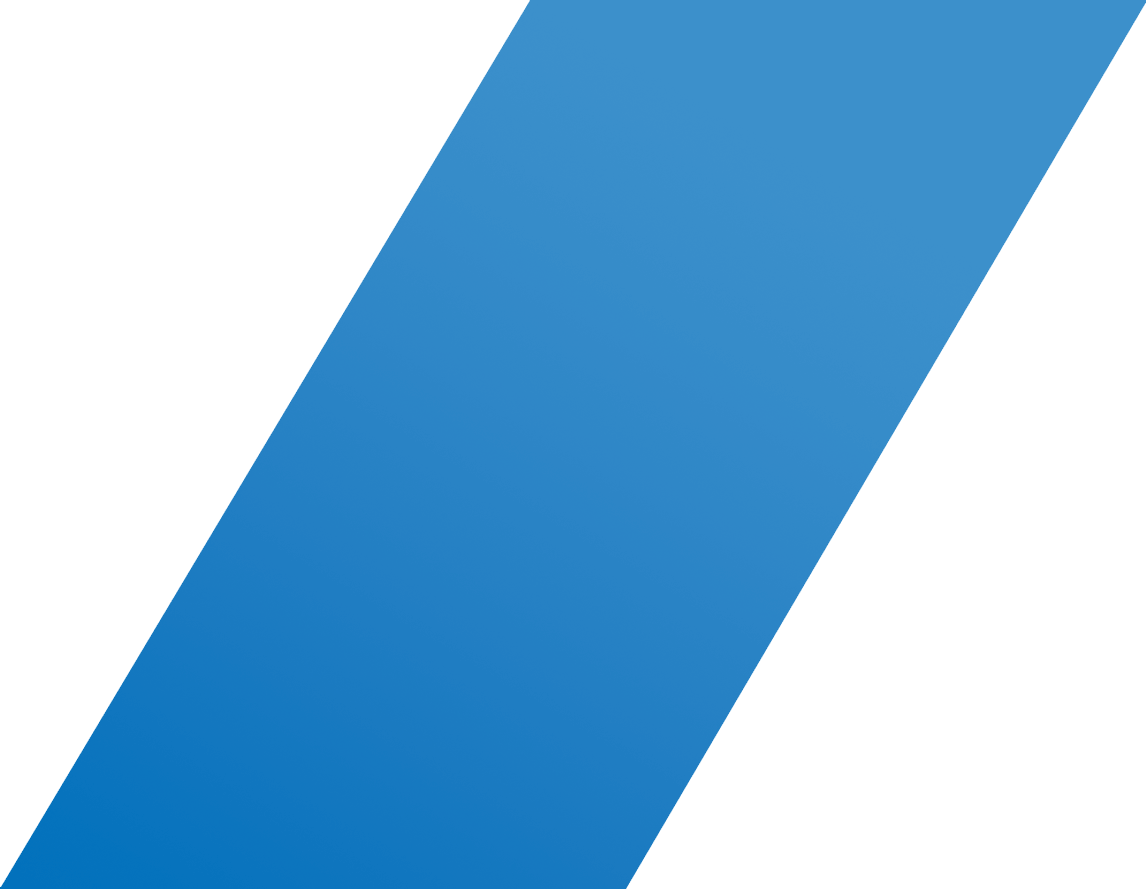
The best way to start your career is to tackle an existing problem with the support of some of the best and brightest minds in the field. Our summer internship is a great opportunity to get valuable, first-hand experience by working on interesting projects related to computer vision and machine learning in one of our divisions – Automotive Division or Face Technology Division.
On this page, you can see an overview of past internship topics that have been successfully tackled by our interns. To stay up to date with upcoming internship opportunities, follow us on Facebook and LinkedIn.
AUTOMOTIVE DIVISION
Internship 2023
Internship 2022
Internship 2021
Internship 2020
Our Automotive Division collaborates exclusively with Qualcomm, developing algorithms for ADAS (Advanced Driver Assistance System). The system detects and tracks objects such as other vehicles and pedestrians, with applications such as automatic braking, lane-keeping assistance, adaptive cruise control, etc.
As we want our CNN network to perform well on diverse number of scenarios, we need data. As collection and annotation is hard and expensive, many scenarios are not covered by it. To get around this we could generate very specific scenarios to train our CNNs. One way to generate these specific scenarios would be using SESAME network, which can translate semantic maps, which carry pixel-wise information, to realistic looking images. The purpose of this internship is to implement such a network and examine how realistic are the car driving scenarios generated using this network.
Prerequisites: Deep Learning basics, Neural Networks, Python
The goal of this task is to compare sigma point methods like unscented Kalman filter (UKF) and cubature Kalman filter (CKF). The idea is to compare different methods in visual tracking use case as it would be interesting to see pros and cons of choosing sigma points differently. After understanding difference between UKF and CKF, implementation of square root variants to get numerical stability will be examined. To sum up, this task would first include implementation of UKF and then modifying it to CKF. Afterwards, implementing their square root variants and running comparison simulations. Finally, if time permits, implementation of other sigma points can be examined.
Prerequisites: Python (C/C++ is also acceptable), basic knowledge of tracking and Kalman Filter
Adverse weather conditions degrade image quality which causes our convolutional neural network to produce erroneous information that is relayed to other car subsystems. In order to properly evaluate the existing prediction quality model in these atypical scenarios, we need to have a way to get more soiling data than we already have. One approach that is considered in the modern automotive industry is to generate synthetic camera soiling scenarios out of existing footage.
Diffusion models are a relatively new approach to image generation whose performance appears to be comparable to the already established GAN model family, while possessing qualitative properties which make training and evaluation easier. Notable examples include OpenAI’s Dall-E 2, Stability AI’s Stable Diffusion, and Google’s Imagen.
The purpose of this internship is to get familiar with the diffusion model family, data preprocessing, data augmentation and model design. The main goal is to utilize a diffusion model for generating new soiled images using publicly available data. During this period, candidates will learn how to train diffusion models, evaluate them quantitatively and qualitatively and compare them with existing generative approaches in the literature.
Prerequisites: Python, Tensorflow, Deep Learning Basics, Neural Networks
Acquiring enough annotated data of certain traffic scenarios is difficult (sometimes borderline impossible, e.g. a cutting in a fire truck on a highway). Creating those scenarios in a simulator and rendering a realistic version of them using a CycleGAN would save a lot of trouble. CycleGANs have shown that they provide great results when trained on unpaired examples which makes them suitable here. The goal of the internship is to train a CycleGAN to render realistic images of predefined rare traffic situations.
Pedestrian detection requires a lot of data, and the process of collecting and labeling data can be very cumbersome and time-consuming. One way to address this issue is to develop a General Adversarial Network that will generate labeled pedestrian data and then add that data to the training data of pedestrian detector. The purpose of this internship is to implement such a network and show that it can be used to improve pedestrian detection performance.
Visual Transformers are a fast-growing model architecture as of late in the field of Computer vision. The purpose of this internship is to train a visual transformer (or use an existing transformer available online as open source) and use it as a baseline to try and make it more efficient without hurting the performance. The focus will be on a non-dense tasks such as image recognition to facilitate the necessary setup and simplify the reuse of open-source resources.
A car, while driving, collects disparity images from its camera (stereo or mono with CNN). The goal of this internship is to implement an algorithm that will merge disparity data from different frames to get more accurate disparity data. The algorithm will involve matching similar points and running optimization on points in the field of view. The key part of the algorithm is removing redundant points from the field of view.
Road lane detection systems play a crucial role in the context of Advanced Driver Assistance Systems (ADASs) and autonomous driving. Such systems can lessen road accidents and increase driving safety by alerting the driver in risky traffic situations. Additionally, the detection of lanes with their left and right boundaries along with the recognition of their types is of great importance as they provide contextual information. Lane detection is a challenging problem since road conditions and illumination vary while driving. The goal of the internship is to investigate the use of a CNN-based regression method for detecting road lane boundaries. Additionally, lane classification needs to be performed to categorize previously detected lane boundaries.
The Kalman filter is an optimal state estimator if we assume that errors have a normal (Gaussian) distribution, and the dynamic model and measurement model are both linear. The dynamic model of interest is a car, for which in every step we have a measurement from the vision camera. The dynamic model of the system is not linear which requires a nonlinear state estimation technique to be used. The most common nonlinear state estimation algorithms are EKF (Extended Kalman Filter), UKF (Unscented Kalman Filter), and Particle Filter. The goal of this task is to compare the EKF and Particle filter state estimation accuracies. The EKF results are already available, while the particle filter implementation needs to be done to compare the mentioned results.
Accumulating a large amount of data is hard to come by. With improvements in photo-realistic simulation, using artificially generated data should be considered. This topic should investigate how the training on such data will translate to the real world. In order to do that, the idea is to generate an adequate amount of artificial data and use it to train a CNN. For evaluation of its performance, both artificial data and open-source real-world data will be used.
Human motion modeling is a continuous problem in the area of computer vision. Different approaches to estimating movement were made such as linear approximation, Kalman Filters (EKF and UKF), neural networks, etc. The purpose of this internship is to improve the performance of an existing multiple object tracking model by adding a neural network as a motion model extension.
Automatic emergency braking (AEB) is a staple of modern advanced driver-assistance systems. Its purpose is to mitigate crashes by initiating braking automatically when hazardous conditions arise. The purpose of this project is to explore the existing AEB algorithms in the available body of scientific work and to develop a custom algorithm and testing environment evaluating the potential for AEB activation on a given dataset and its resimulations given different changes in the object detection and tracking pipeline. The solution is expected to blend seamlessly with the existing environments using the existing resimulation pipelines and build systems.
Adverse weather conditions such as rain or snow can have a significant impact on autonomous driving performance. At the same time, such data can often be difficult to obtain. Therefore, advanced data augmentation techniques can be used to expand existing datasets. One of the newer approaches is to use a generative adversarial network (GAN) to generate synthetic data. The goal of this project is to develop a GAN for generating adverse weather condition scenarios.
Convolutional neural networks proved to be very effective for image processing, and are increasingly being used for sound processing. Indeed, by computing spectrograms of audio signals, we obtain visual representations of their spectrum of frequencies. These images can then be readily used in CNNs. The goal of the project is to make a brief review of the literature, implement a CNN for sound classification for an application of choice, and experiment with different hyperparameters, particularly those specific to audio data.
Data collection and data marking are usually done manually and can prove to be a tedious, expansive and error-prone process. In order to increase the amount of data and improve robustness of a dataset, artificial data approaches can be used. The goal of this internship project is to use generative adversarial network (GAN) to generate usable artificial data. The student will learn how to implement GAN, train GAN on in-house dataset and have an opportunity to further investigate different models and approaches to improve results. Prior experience in the field of machine learning is preferable, but not mandatory. The estimated duration of this project is six to eight weeks.
Consider developing a supervised learning method with data stemming from a video, for instance a forward-looking camera for an autonomous vehicle. It is guaranteed that many training samples coming from consecutive frames of a video will be too similar. Such (almost) repetitive samples may lead to overfitting, while at the same time significantly increasing training time. The goal of this internship project is to develop a preprocessing step to detect and remove such samples. The task can and will be tackled in different ways, including “conventional” methods and ML algorithms, and gradually progressing from simpler to more complex formulations of the problem.
This topic is in the area of object tracking applied in automotive industry. During the internship period, the student will get an insight into modelling a complex system behaviour such as behaviour of a vehicle as well as how to utilize Kalman filter for object tracking. Furthermore, the student will gain practical knowledge with implementation of Extended Kalman Filter, Unscented Kalman filter, and, time allowing, introduction of state constraints in Kalman filtering. Knowledge of estimation theory as well as basic understanding of linear algebra are needed to successfully finish this project. State constraints are an interesting topic and successfully implemented in model predictive control, but there are also articles that suggest that they can be used with EKF and UKF as well. During this internship, it is encouraged to investigate approaches how to do it, with an input from mentor, and try to implement it in UKF. If the work proves engaging and estimation of work inadequate, the internship can be extended for two more weeks. However, if for any reason the implementation cannot be done, just getting an insight in state constraints with Kalman filters would be considered beneficial.
Obtaining real world data needed to train a deep neural network is usually a challenging, costly and time consuming task. The most labor intensive part is manually marking a large amount of data for supervised learning. One way to overcome this issue is to create synthetic data that can be automatically marked using a game engine. The goal of this project will be to create a rather innovative synthetic “domain randomized” dataset similar to the one described in this article that can be used to train a traffic light recognition CNN.
This document presents an internship project developed for Visage Technologies AD. The estimated duration of this project is six to eight week. During this period it is expected that a student will get a brief picture about vision system for vehicles, understand convolution neural networks (CNNs), how to design them, go through the learning process and analyse the output. A student should be able to read a scientific paper and get a critical overview of the work. The practical part of the project includes implementing CNN for mono depth, running training on public data set (e.g. KITTI), tuning hyper-parameters analysing results. To successfully finish the project, a student has to have good programmer skill and some understanding of machine learning. Knowledge about deep learning and CNN would be preferable, but it is not mandatory. Our mentors will be there to give you advice and discuss the literature. They will also help you organise your work and give you some useful tips through the review process.
FACE TECHNOLOGY DIVISION
2023
2022
Our Face Technology Division develops cutting-edge face and beauty AR technology. We are proud owners of visage|SDK and makeup|SDK, powerful and 100% proprietary software development kits. These SDKs provide top-notch face tracking, analysis, and recognition, and enable unique AR makeup experiences.
Synthetic data offers many useful features when it comes to training machine learning models. However, a drawback is the possibility of generating unrealistic samples, which can hinder the model’s ability to transfer learned knowledge to real-world scenarios. The goal of this internship is to address this issue by applying a process that degrades the quality of an existing render of a person (synthetic image) and then using a generative model to restore the image. As a results, we hope to obtain a realistic image of a person, while still being able to reuse the original annotations such as face keypoints and bounding boxes.
Prerequisites: Python, Basic knowledge of deep learning
Photorealistic human face rendering will be investigated to generate synthetic face data. Data generation should be implemented using Unity with parametrization of head position, eye gaze, and facial expression. A final goal is to check if synthetic face data could be used in workflow for testing face tracking software. Known parameters and annotations in the face data would be compared to those predicted by the visage|SDK.
Prerequisites: Unity basics, C#
GANs have revolutionized the fields of photorealistic image synthesis, image-to-image translation and style transfer. The research community is now mainly focused on controlling different aspects of image synthesis using GANs. Join us in discovering how to use the CA-GAN model to modify the colors of a specific face area (e.g. lips) and transfer them to another image while keeping the rest of the image intact. The goal of this project is to set up a training framework with the CA-GAN model and train it in a weakly supervised manner.
Work on introducing new features to a fun game that utilizes your facial movements, expressions, and emotions to smash targets and score points. It is an exciting opportunity to code a new game logic, as well as introduce new features and graphics.
We publish new internship topics every summer – follow us on social media to stay up-to date. We also invite you to check out other interesting career opportunities.
This website uses cookies to provide you with the best possible user experience. You can adjust your cookie preferences here. To learn more, please read our Privacy notice.
Marketing cookies are used by third parties like Facebook, Google, and LinkedIn to track how you use our website and deliver more relevant ads.
If you disable this cookie, we will not be able to save your preferences. This means that every time you visit this website, you will need to enable or disable cookies again.
Performance cookies, preference cookies, and other unclassified cookies are used to optimize your user experience of the website.
Strictly Necessary Cookies are necessary for the website’s functioning and you accept them if you want to use this Website.
Statistical cookies give us insights into how people use our website. They collect and report data anonymously to help us improve your experience without invading your privacy.
Strictly Necessary Cookies are necessary for the website’s functioning and you accept them if you want to use this Website.