FACE TECHNOLOGY DIVISION
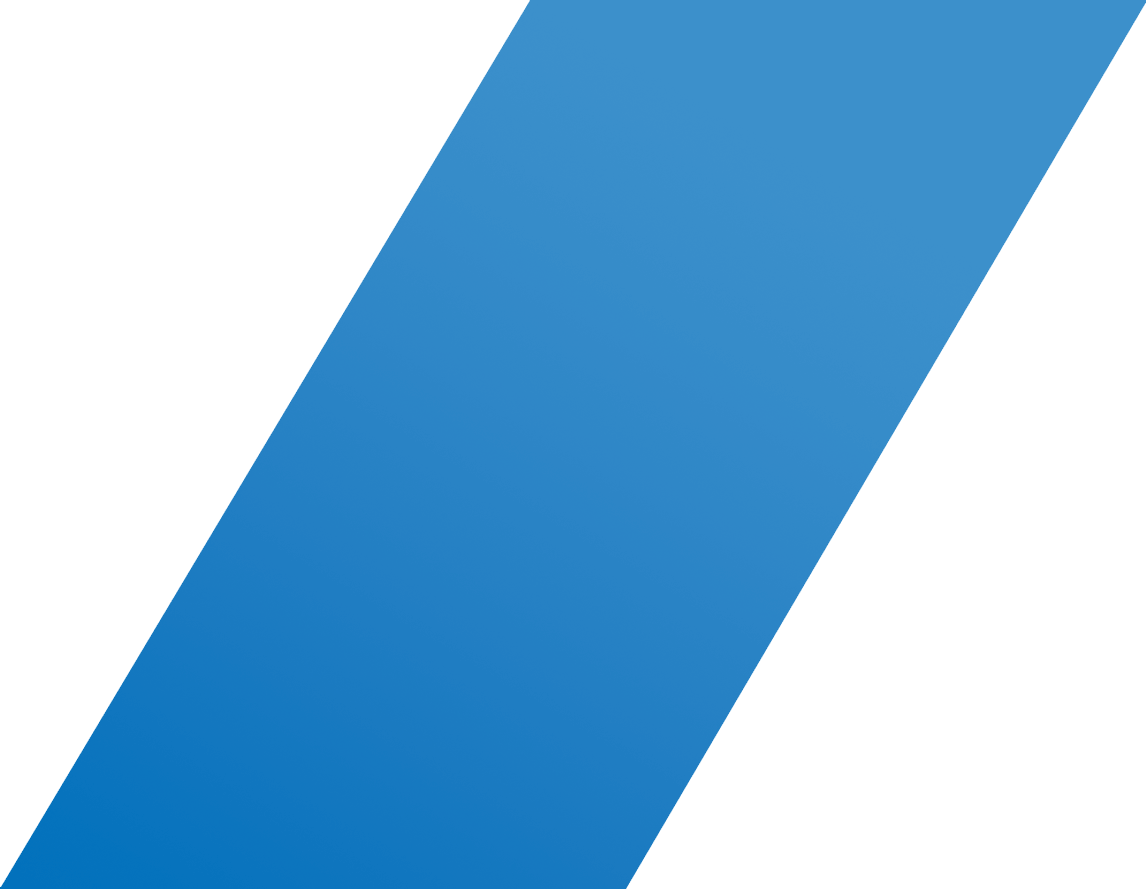
The best way to start a career is to learn from some of the best and brightest minds in the field. So, come spend an unforgettable summer with us and gain priceless first-hand experience by working on exciting projects.
If you are a Master’s student or you’ll become one this fall, this internship was designed for you. Gain hands-on experience with the possibility of permanent employment after your graduation, and an outstanding reference for your resume.
The internship will be held in our Zagreb office for 6 weeks (between the end of August and the beginning of October). It is a full-time, financially compensated position that lets you experience what it’s like being a part of a successful company.
Choose one of the available topics and collaborate with our experienced engineers. New ideas and curiosity are always welcome – we want you to grow professionally, but also appreciate the fresh perspectives you bring to the table.
Choose your internship project
At Visage Technologies, we have two separate divisions – Face Technology Division and Automotive Division. Learn more about each division and available projects below, and apply before June 2nd.
Our Face Technology Division develops cutting-edge face and beauty AR technology. We are proud owners of visage|SDK and makeup|SDK, powerful and 100% proprietary software development kits. These SDKs provide top-notch face tracking, analysis, and recognition, and enable unique AR makeup experiences.
Our Automotive Division collaborates exclusively with Qualcomm, developing algorithms for ADAS (Advanced Driver Assistance System). The system detects and tracks objects such as other vehicles and pedestrians, with applications such as automatic braking, lane-keeping assistance, adaptive cruise control, etc.
“A student internship at Visage Technologies is a kind of internship that I would highly recommend to every student who wants to gain some real-world experience in the automotive or facial recognition industry. The task you work on is applicable, complete, and challenging, yet there is enough time and opportunity to make new acquaintances and meet the company and its way of working. With the guidance and support of mentor(s), you can learn some valuable tips and deepen your skills.”
“My internship was an enriching experience, marked by a welcoming and collaborative environment. The team was always open for discussions and the mentors were exceptional—genuinely great people who always steered me in the right direction. It struck the perfect balance between professional development and enjoyment, making it not just productive but also fun.
For those who are passionate about machine learning and computer vision, this internship is a perfect match, blending professional guidance with hands-on experience in a friendly and open atmosphere.”
“During my internship, I was able to meet many great people. Every week there was a cake or something sweet in living room, you could play 8 ball pool and workout whenever you wanted. Despite all of that, everyone was working really hard. The working atmosphere is excellent! That month and a half really flew by quickly. I had a great time and learned a lot. 9 months since the internship started, I’m still part of Visage Technologies team as a student developer and I’ll soon become a full time employee. If you’re interested in autonomous driving or computer vision, I would definitely recommend this company and its internship program.”
“Last year, I worked on the topic “Learning from Image – CNN & MonoDepth” in which the ultimate goal was to develop a deep convolutional model (CNN) for depth estimation. Solving this problem was very interesting and I learned a lot. The atmosphere in office was great – working and motivating, but with fikas (coffee and cookies breaks) and billiard game breaks.”
“I would recommend the student internship at Visage Technologies to anyone interested in Research and Development as the focus of the internship is work on a research topic with a constant support of an experienced mentor. Additionally, I want to point out that the atmosphere is truly fantastic; everyone is very kind and helpful thus integrating you well in the work environment of the company.”